The Basics of Machine Learning Operations in Power Spot Trading
energy-trading
10 Aug 2023
Sourabh Raj, Head of Data Science
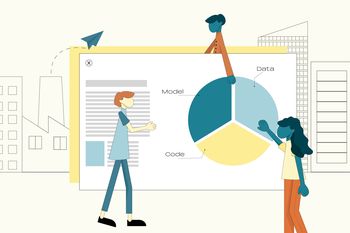
Systematic trading in energy spot markets is backed by machine learning-based trading models that spot prespecified patterns in incoming data and output signals, which trigger buy or sell decisions.
Taking trading models to production isn't a walk in the park: technical teams should have three components in place — a model itself, data and code that glues them together — to go live.
Therefore, it's necessary to establish the right deployment strategy that will encompass machine learning operations (MLOps) which are the main focus of this article.
Common MLOps Practices and Their Application in Power Spot Trading
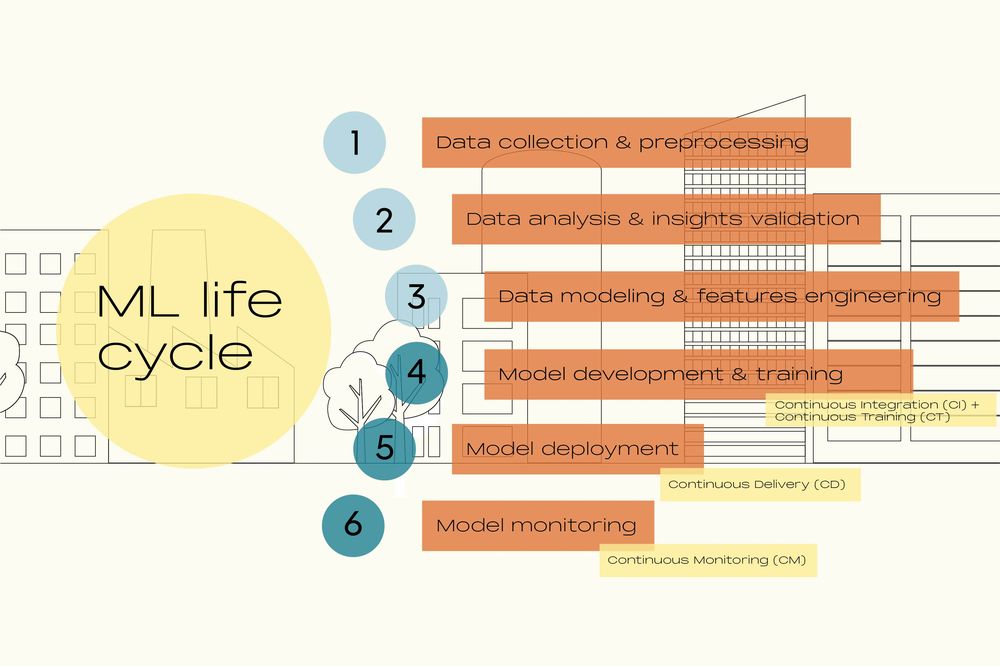
MLOps refer to practices and processes that accelerate and facilitate the entire machine learning lifecycle, in particular deployment, monitoring and maintenance of trading models in production environments.
MLOps bring together a team of analysts, data scientists and data engineers alongside MLOps engineers who take the best from multiple software development practices and tailor them to a trading model's life cycle.
Continuous integration (CI) of code submitted by each contributor — including automated code testing and validation — to catch any code errors or inconsistencies and facilitate continuous updates rollout
Continuous delivery (CD) of an updated trading model to selected production-like environments in a controlled, systematic and automated manner
Continuous training (CT) of a model in production environments to account for any change in patterns and stay accurate and effective over time
Continuous monitoring (CM) of model's performance, explainability, distribution of data and conformance with risk metrics
Therefore, technical teams can apply one, several or all MLOps principles from the above to automate the life cycle of a trading model.
ML Life Cycle Automation: Levels of Maturity
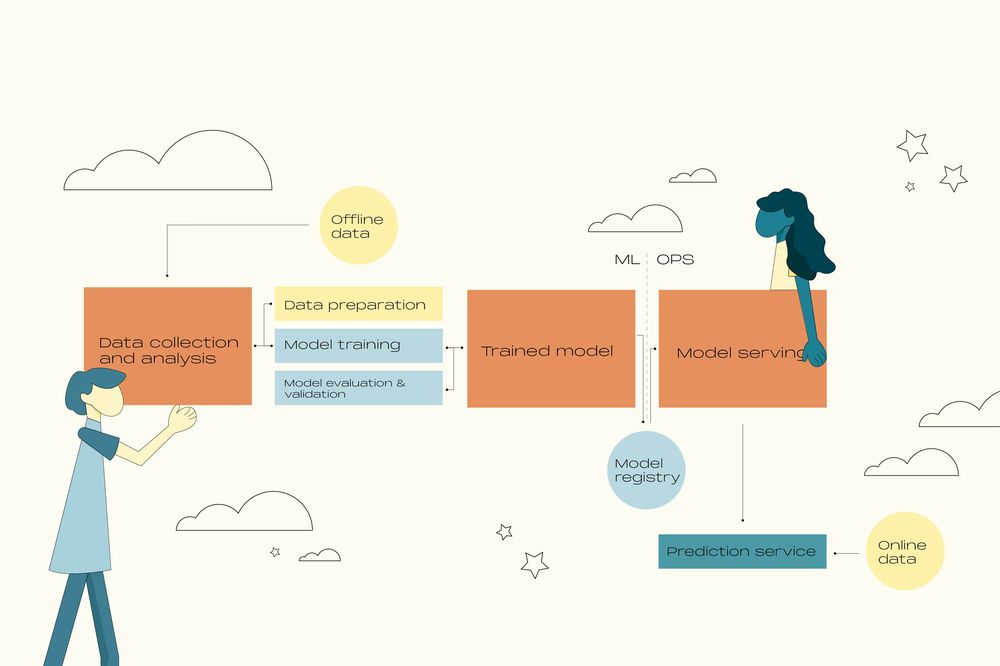
The degree of ML life cycle automation varies across the power industry from manual processes to end-to-end machine learning pipelines.
The higher the level of automation, the more mature the ML processes that increase the overall velocity of the model's training.
Level 0: manual. At this level, human intervention is required at every stage of a model's life cycle as data processing, model development, training, deployment and monitoring are performed manually. Manual execution typically involves running scripts or notebooks without a systematic workflow — and therefore is tedious and susceptible to errors.
Level 1: automated scripts. Basic automation of repetitive tasks like data processing, model training or evaluation is introduced by applying scripts or command-line tools. However, the pipeline components remain largely separate — a unified framework that accommodates the entire pipeline might not yet exist.
Level 2: workflow management. Workflow management tools or frameworks — e.g. Apache Airflow or Kubeflow Pipelines — are employed to orchestrate the different stages of the ML pipeline. Better team coordination and task scheduling enable parallelism and dependency management. This increases the overall pipeline efficiency and facilitates the definition of the sequence of stages and their interdependencies.
Level 3: automation with model versioning. In addition to workflow management, ML model versioning tools — e.g. MLflow or DVC — are introduced at the third level to efficiently track model versions, associated code and metadata. This enables reproducibility and facilitates model comparison and selection of the best-performing models for deployment.
Level 4: introduction of continuous integration/continuous delivery (CI/CD). At this level, CI/CD practices are integrated into the ML pipeline. Version control systems — e.g. Git — are combined with automated testing frameworks to allow for continuous integration, where code changes and model updates are regularly merged, validated and tested. Continuous delivery reduces manual intervention and accelerates the process by automatically deploying validated models to production.
Level 5: full automation. The highest level of pipeline automation involves not only end-to-end automation but also continuous monitoring of the deployed models. Monitoring tools — e.g. EvidentlyAI or nannyML — are employed to track model performance, detect concept drift and trigger alerts or retraining when necessary.
Let's refer to the following example to understand how a trading company can automate the machine learning life cycle by combining Gitlab, MLFlow, Docker, AWS and Airflow:
The technical team utilizes either Jupyter Notebook or Python scripts to manipulate fundamental and historical data, extract relevant features and conduct model experiments. Simultaneously, proper model tracking and version control are covered by GitLab and MLFlow.
Once the trading model is ready for deployment, data scientists can initiate a CI/CD pipeline for automated testing and containerization through Docker. On creating a Docker image with all the necessary dependencies, libraries and configurations, the model can be seamlessly deployed across various platforms.
When the Docker image is pushed to the container registry, the deployment process is automated and executed on-premise or via AWS cloud infrastructure. A/B testing is integrated into the CI/CD workflow to compare different model versions effectively. Moreover, Airflow is employed to facilitate continuous training in a production environment.